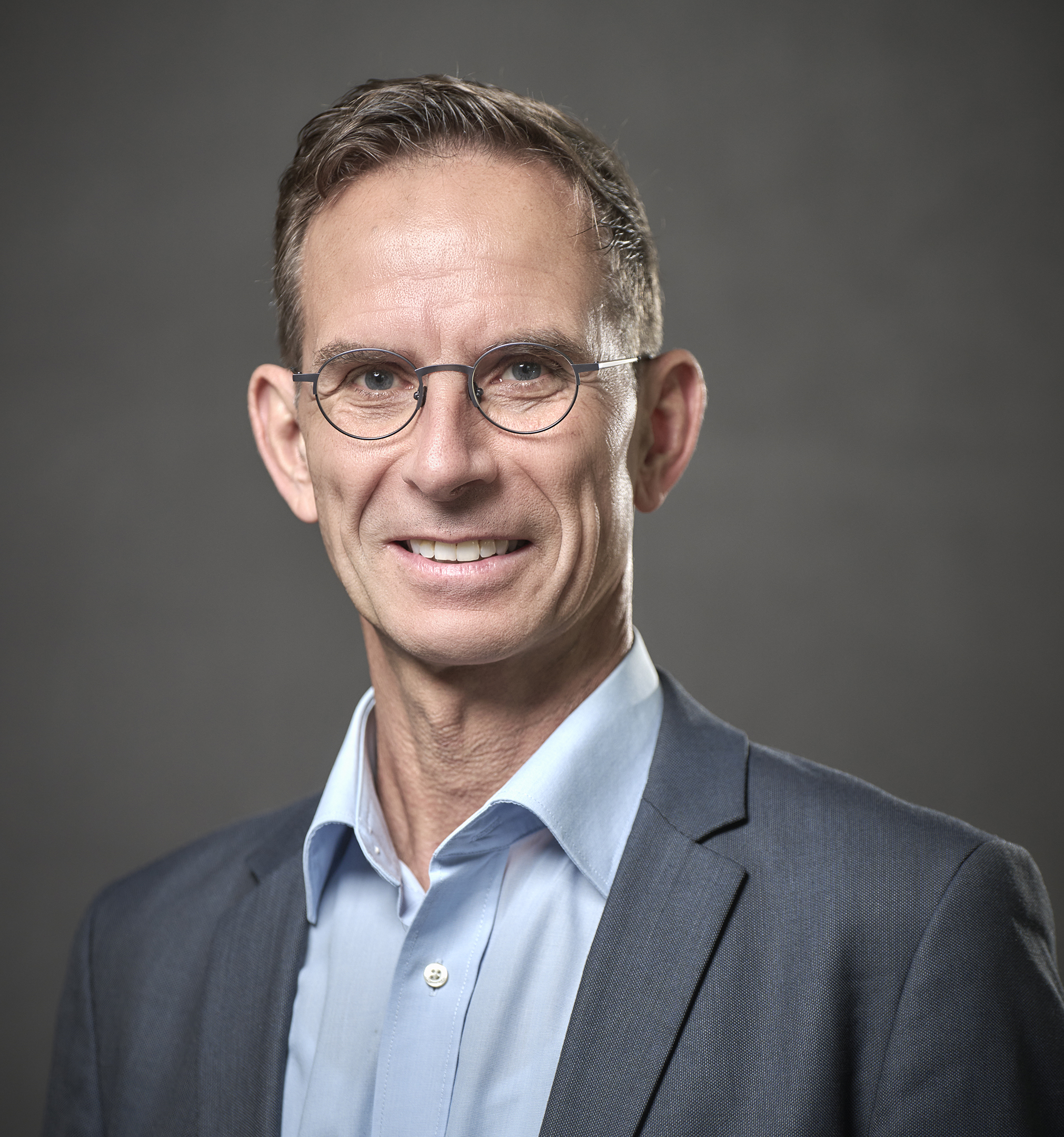
Prof. Dr. Torsten Braun - University of Bern, Switzerland
Title: Federated and Split Machine Learning in the Internet of Things
Abstract: In this talk we discuss approaches for distributed machine learning (ML) in resource-constrained edge-supported Internet of Things (IoT) networks. Federated Learning (FL) and Split Learning (SL) are popular approaches in such wireless edge networks. First, we present Early Exit of Communication (EEoC), which adaptively splits ML inference in an IoT edge computing environment to meet latency and energy constraints. This layer-based (vertically partitioned) approach has been extended by Distributed Micro-Split Deep Learning in Heterogeneous Dynamic IoT (DISNET), which adds horizontal partitioning to better support flexible, distributed, and parallel execution of neural network models on heterogeneous IoT devices under dynamic conditions. Then, we also consider the training aspect by developing and evaluating Adaptive REsource-aware Split-learning (ARES), a scheme for efficient model training in IoT systems. Recent work suggests Dynamic FL (DFL) for heterogeneous IoT, which uses resource-aware SL and FL based on similarity-based layer-wise model aggregation.
Bio: Prof. Dr. Torsten Braun is head of the Communication and Distributed Systems (CDS) research group at the Institute of Computer Science, University of Bern, where he has been a full professor since 1998. He got the Ph.D. degree from University of Karlsruhe (Germany) in 1993. From 1994 to 1995, he was a guest scientist at INRIA Sophia-Antipolis (France). From 1995 to 1997, he worked at the IBM European Networking Centre Heidelberg (Germany) as a project leader and senior consultant. He has been a vice president of the SWITCH (Swiss Research and Education Network Provider) Foundation from 2011 to 2019. He has been a Director of the Institute of Computer Science and Applied Mathematics at University of Bern between 2007 and 2011, and from 2019 to 2021.
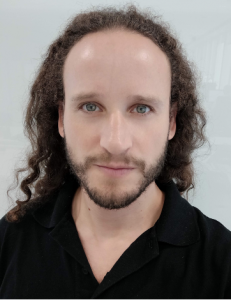
Dr. Nicolas Kourtellis - Telefónica, Spain
Title: Powering the Next Generation Telco Networks with Privacy-Preserving AI
Abstract: As faster, more complex networks (5G, 6G, etc.) emerge, traditional telco network management solutions are reaching their limits, with respect to AI model performance, security and privacy protection, and fairness offered. At Telefonica, we explore cutting-edge methods using cloud infrastructure, hierarchical network structures, edge and end-user computing, and privacy-preserving AI models. In this talk, we will cover our recent efforts to develop secure, private, fair, and scalable systems to meet the demands of next-gen networks and applications.
Bio: Dr. Nicolas Kourtellis is Head of the Systems AI Lab (SAIL) and Co-Director of Telefonica Research, a 25+ researcher team based in Barcelona, Spain. He holds a PhD in Computer Science & Engineering from the University of South Florida, USA (2012) and has over 90 published peer-reviewed papers and 8 filed patents. Currently, he focuses on privacy-preserving AI and federated learning on the edge, modeling/detecting with AI user online privacy leaks, as well as inappropriate/fraudulent behavior on social media. He executed several research visits in ANL (USA), INRIA (France), Yahoo (Spain/USA), UCL (UK), CUT (Cyprus), AUTH (Greece), FORTH (Greece), and others. He has served in many technical committees of top conferences and journals, and presented his work in top academic and industrial venues including Mobile World Congress 2021 and 2023, Apache Foundation, etc. His work has been covered by major news outlets such as Nature, New York Times, The Atlantic, New Scientist, Washington Post, Wired, and others. In 2022, he was ranked among the World’s Top 2% Scientists (2021) in the list prepared by Elsevier BV, Stanford University, USA.